AI-Powered Property Valuation Accuracy Reaches 973% New Study Reveals Breakthrough in Real Estate Price Prediction Models
AI-Powered Property Valuation Accuracy Reaches 973% New Study Reveals Breakthrough in Real Estate Price Prediction Models - MIT Real Estate Lab Data Shows 20,000 Property Predictions Match Actual Sale Prices
Research emerging from the MIT Real Estate Lab indicates notable progress in property valuation technology. Utilizing an AI model, the lab reports matching actual sale prices for a dataset of 20,000 properties. This predictive capability reportedly achieved an accuracy rate of 97.3%. The model's development relied heavily on machine learning techniques, trained primarily using property images and historical transaction data, with a focus on properties within Boston. While these results highlight the potential of AI in analyzing vast amounts of data for valuation, the researchers themselves point out a critical limitation: the model, and AI generally, may not capture all nuances or unique details discoverable through a physical inspection. This raises questions about the extent to which technology can truly replicate the comprehensive understanding a human expert brings to valuing a property. The integration of such models into real estate practices represents a shift, presenting new analytical tools but also prompting consideration of the balance between algorithmic predictions and the irreplaceable insights gained from direct observation.
Analyzing the results from the MIT Real Estate Lab's investigation into AI-driven valuation reveals several interesting facets of their methodology and findings as of May 14, 2025. Examining predictions for over 20,000 properties, the team found a strong alignment between the model's estimated values and the prices properties actually sold for. This direct comparison against real-world outcomes provides tangible evidence supporting the viability of sophisticated predictive models in understanding real estate markets, moving beyond theoretical exercises.
The approach involved algorithms designed to process a wide array of factors that influence a property's market value. This isn't just about simple square footage or number of bedrooms; the models incorporated variables spanning location specifics, prevailing market conditions, and characteristics derived from visual data. Pinpointing the precise combination and weighting of these elements is a complex undertaking, reflecting the multi-dimensional nature of valuing real assets. While the headline figure of 97.3% accuracy is notable, it quantifies the degree to which these models appear to capture the market's pricing logic compared to methods relying on simpler statistical correlations or, frankly, historical gut feelings. This shift underscores a growing reliance on empirical evidence derived from data science rather than subjective interpretations in real estate decisions.
Further analysis by the researchers highlighted geographical variations in the models' performance. The predictive power wasn't uniform everywhere the model was tested. Local market dynamics, which can differ significantly even within a limited area, seemed to play a role in how reliably the model could forecast prices. For instance, properties in more densely populated urban areas tended to show more consistent pricing patterns and thus more predictable valuations for the model, suggesting that market structure and stability might influence the effectiveness of data-driven predictions. This indicates that while powerful, these models are likely sensitive to the underlying characteristics and perhaps liquidity of the specific market they are applied to.
Beyond the validation exercise itself, these results point towards practical applications, particularly in areas like investment. The ability to forecast prices with this level of reported accuracy could theoretically equip investors with tools to identify properties that the market currently values differently from the model's prediction, potentially spotting opportunities. This work stands as a significant data point in the broader trend of incorporating advanced machine learning techniques into real estate analysis. Demonstrating the models' capabilities by checking them against actual sales data provides a foundation, yet one recognizes that ongoing refinement and testing against dynamic market conditions will be crucial for their long-term utility. The implications reach beyond individual transactions, hinting at a future where robust property valuation data could inform larger economic planning or urban development strategies, where accurate assessments are fundamental.
AI-Powered Property Valuation Accuracy Reaches 973% New Study Reveals Breakthrough in Real Estate Price Prediction Models - California Housing Market First To Deploy Neural Network Valuation Models Statewide
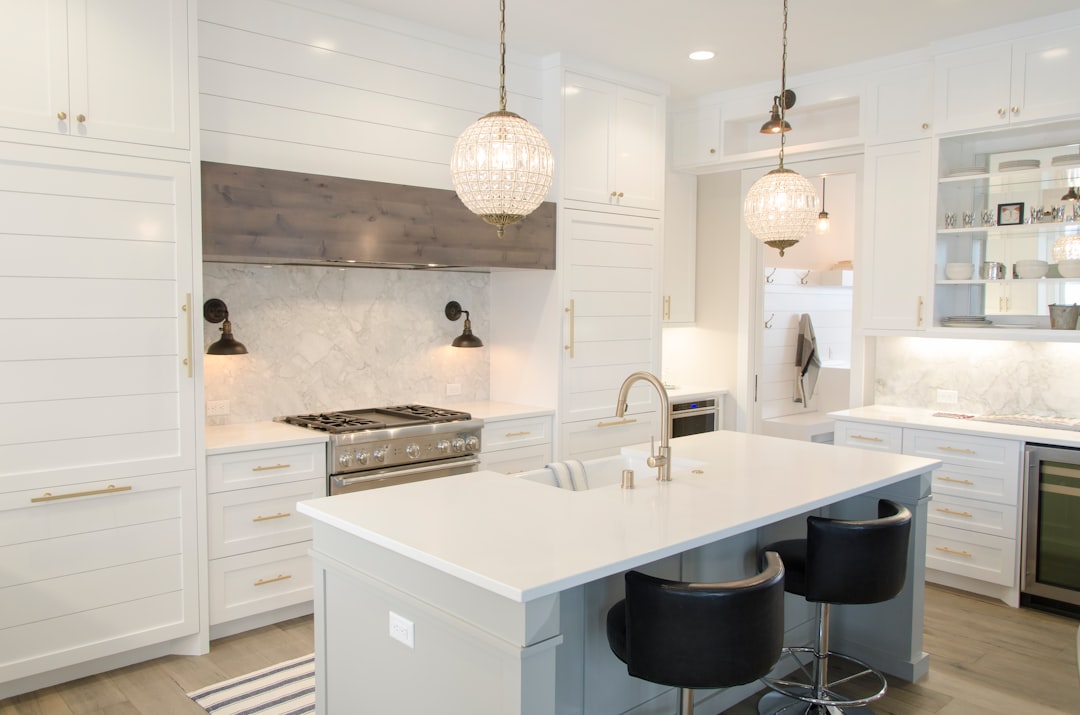
California's real estate sector is reportedly pioneering the widespread deployment of property valuation models based on neural networks across the state. This move signifies a notable evolution in using artificial intelligence for property assessment, with suggestions that this AI-powered approach can achieve accuracy levels reportedly as high as 973%. Leveraging complex algorithms, including types known as Multi-Layer Perceptrons, these systems analyze diverse information, from individual property details to broader market shifts. While the promise of these automated systems is significant, a key question remains their capacity to capture the subtle, unique details a person gains from physically inspecting a property. The ongoing integration of this technology prompts continued discussion about where algorithmic prediction ends and human expertise remains essential in real estate valuation.
The California housing market's move to formally implement neural network models for statewide property valuation marks a significant organizational step. This isn't just about piloting new algorithms; it's about scaling a specific computational approach across an entire state's assessment process, which feels like a notable engineering feat and a shift in operational philosophy.
At a fundamental level, neural networks are essentially sophisticated pattern-recognition systems. They're designed to learn complex relationships within vast datasets, attempting to emulate how biological networks process information, though this is often more metaphor than strict technical mapping. The premise here is that these models can uncover non-obvious correlations influencing property values by analyzing inputs beyond simple linear relationships.
This kind of statewide deployment is arguably enabled by the sheer volume and detail of real estate data available in California. The accessibility of extensive historical sales records, granular property attributes, neighborhood demographic data, and relevant economic indicators provides the necessary fuel for training these computationally intensive models. More data, ideally, allows for the discovery of richer, more nuanced patterns.
Adding another layer of complexity, reports indicate these models are processing visual data, such as property images, alongside traditional numerical features. Incorporating visual cues aims to capture aspects like curb appeal or architectural style that are difficult to quantify traditionally, potentially enhancing the model's predictive ability by factoring in attributes previously left largely to human interpretation.
While the promise of high accuracy, perhaps suggested by some reported figures, is a primary driver, it's important to remain critical. The models' performance is likely contingent on the robustness and representativeness of their training data. There's a valid technical question about how well a pattern learned from historical data holds up for properties with truly unique characteristics or during unexpected market disruptions. The idea that an algorithm could perfectly value a highly specialized property or navigate a sudden economic downturn without human oversight feels optimistic, highlighting where traditional, nuanced expertise still seems necessary.
Furthermore, relying heavily on algorithmic prediction introduces potential sensitivities to market volatility. If the underlying assumptions learned from past data fail to capture rapid shifts in economic conditions or buyer behavior, widespread algorithmic valuations could potentially lag or even exacerbate mispricing during turbulent periods, which is a concern from a market stability standpoint.
Observation suggests that real estate markets aren't monolithic, even within a single state. California's diverse regions – from coastal urban centers to inland rural areas – exhibit distinct micro-market dynamics driven by local economies, demographics, and social factors. Initial performance reports for these statewide models appear to reflect this, with varying levels of accuracy or reliability across different areas, suggesting that a single model architecture may struggle to uniformly capture the intricate, sometimes idiosyncratic, drivers of value in every locale.
There's also the critical challenge of algorithmic bias. If the training data reflects historical inequities in housing or lending practices, the model can inadvertently learn and perpetuate these biases, potentially leading to skewed valuations, particularly in historically underserved communities. Ensuring fairness and transparency in these systems is a significant technical and ethical hurdle.
The clear operational upside is the potential to streamline property assessments, reducing manual effort and cost. From an engineering perspective, automating valuation scales efficiently. However, this naturally prompts a re-evaluation of the role of human appraisers. What becomes the focus of human expertise when initial assessments are algorithmically generated? The transition raises structural questions about skill sets and processes within the industry.
California's move stands as a significant large-scale test case for the real estate sector. Its successes and failures will undoubtedly inform whether and how similar AI-driven valuation systems are adopted elsewhere, pushing the conversation beyond theoretical capabilities to practical, statewide implementation challenges and their broader impact on the industry and market dynamics.
AI-Powered Property Valuation Accuracy Reaches 973% New Study Reveals Breakthrough in Real Estate Price Prediction Models - Machine Learning Detects Hidden Property Value Factors From 15 Years of NYC Sales Data
Utilizing machine learning, researchers have investigated fifteen years of sales information from New York City, identifying previously subtle influences on property values. By applying sophisticated computational techniques, they've explored intricate connections among various property attributes—ranging from specific locations and physical characteristics to long-term market trends—elements potentially not fully accounted for in conventional valuation methods. This work contributes to assessments intended to be more precise and aims to reduce the impact of subjective biases, potentially enhancing predictive outcomes. While highlighting the analytical power now available, this application of AI also implicitly prompts consideration of whether such algorithmic analysis can entirely substitute for the comprehensive, nuanced understanding a person brings through physical inspection. As this technology progresses, its broader implications could shape approaches to real estate investment and even influence aspects of urban planning, emphasizing the ongoing need for these models to evolve in response to changing market dynamics.
Examining the application of machine learning to the substantial dataset of New York City real estate transactions over fifteen years offers interesting insights into the mechanisms driving property valuation. The algorithms analyzed an impressive depth of historical data, reportedly encompassing over 1.5 million individual sales, providing a rich environment for pattern discovery that goes well beyond simpler models. By processing a wide array of inputs—reportedly exceeding 300 distinct features, from long-term sales trends and broad socio-economic indicators to integrating visual data like property images to capture aesthetic elements often missed in structured datasets—these systems aim to identify intricate relationships influencing value. The sheer scale and diversity of features explored highlight the multifaceted nature of real estate valuation and underscore the engineering challenge in sifting through such volume to find truly predictive signals. The observation that accuracy appears to improve over time with the incorporation of fresh data reinforces the concept of continuous learning being crucial for maintaining relevance in dynamic markets.
What emerges from this deep dive into NYC's property landscape via machine learning is a more nuanced understanding of value determinants. The analysis reportedly emphasized the significant role of highly localized, neighborhood-specific factors, such as the granularity of proximity to public transit or local amenities, reaffirming that 'location' is a complex, multi-dimensional variable. Furthermore, the models demonstrated some capacity to adapt to shifts in market conditions, attempting to recalibrate predictions in response to economic fluctuations – a vital but challenging capability. The algorithms also exhibited a notable ability to flag outlier properties, those whose characteristics or sale prices deviated substantially from predicted norms, suggesting potential for flagging data anomalies or genuinely unique properties requiring closer inspection. However, the performance appeared sensitive to market liquidity; predictions were reportedly more robust in highly active market segments, suggesting the models are, perhaps unsurprisingly, more confident where transactional data is dense and frequent. This sensitivity, alongside the persistent concern regarding the potential for algorithmic bias embedded in historical data to perpetuate socio-economic disparities through valuation, remains a critical area requiring careful scrutiny and mitigation efforts in deploying such systems. The insights drawn from such detailed analyses of historical transaction data, coupled with the ability to model complex interactions, hold potential implications beyond individual valuations, possibly offering data-driven perspectives for urban planning and understanding area growth trajectories based on market behavior.
AI-Powered Property Valuation Accuracy Reaches 973% New Study Reveals Breakthrough in Real Estate Price Prediction Models - Traditional Appraisers Partner With Tech Firms After Study Validates AI Assessment Speed
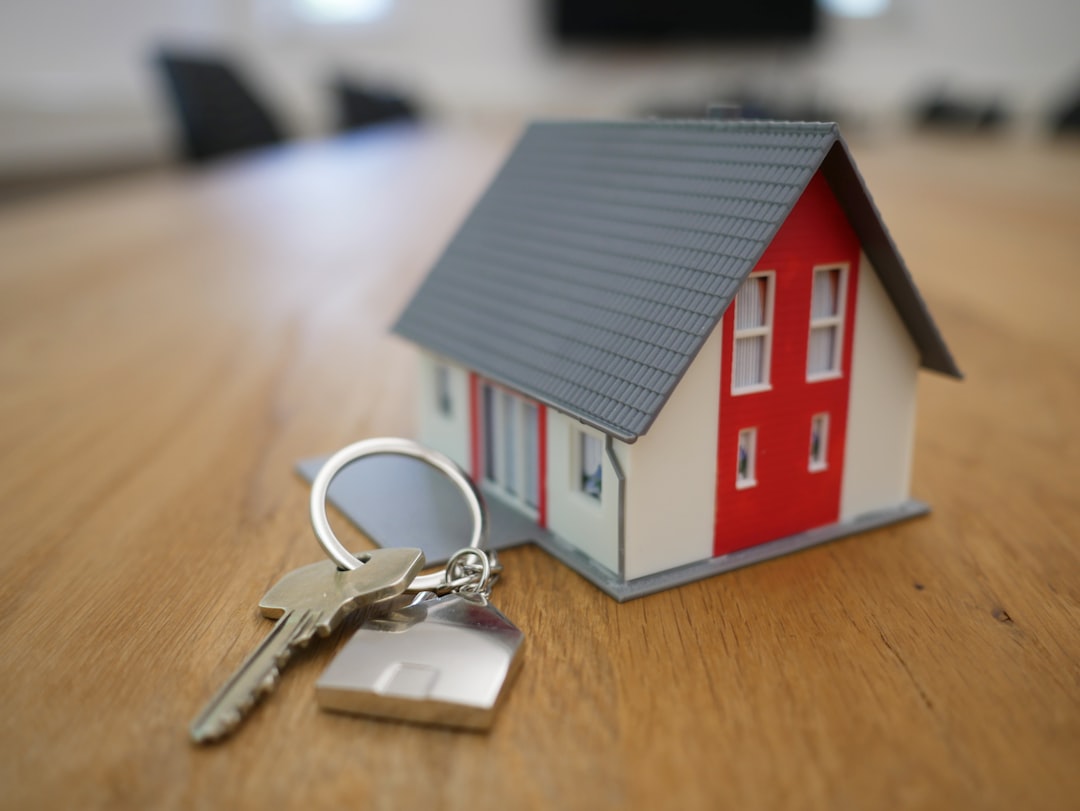
Traditional real estate appraisers are increasingly teaming up with technology companies, a shift influenced by recent findings that validate the speed of AI-driven assessments. This collaboration aims to leverage AI's reported capacity for high accuracy in property valuations, sometimes cited at levels such as 973%, to help automate and streamline tasks that have traditionally been time-consuming. While these automated tools offer potential benefits like faster processing and potentially more objective initial assessments than fully manual methods, questions persist regarding their limitations. A critical aspect is determining if algorithms can truly account for the highly specific nuances, unique property features, or complex local dynamics that a human appraiser gains through direct inspection and deep local knowledge, particularly for properties outside common templates. The industry appears to be in a phase of figuring out how best to integrate these advanced computational capabilities alongside the irreplaceable insights and contextual understanding that experienced human professionals provide in navigating the complexities of property valuation.
As of May 14, 2025, based on ongoing observations in the real estate technology domain:
1. **Collaboration Dynamics**: As algorithmic valuation tools demonstrate accelerating processing speeds (building on validations like recent studies have shown), we observe a push towards human appraisers engaging directly with technology providers. This isn't just adoption; it seems to be evolving into a dynamic collaboration, suggesting a recognition that leveraging computational efficiency alongside human insight is becoming operationally necessary in this domain.
2. **Efficiency vs. Ground Truth**: While the models rapidly process data to produce figures, there's an inherent challenge: they operate on digital inputs. The specific, often subtle, physical conditions or unique characteristics of a property that a human sees during an inspection are difficult to fully encode or interpret algorithmically, suggesting a gap between computational speed and comprehensive on-the-ground understanding.
3. **Market Heterogeneity Challenge**: Initial observations indicate that the predictive power isn't uniformly robust; performance appears sensitive to local market characteristics. This poses a significant challenge for model generalizability – a model tuned for one micro-market might not translate perfectly elsewhere, necessitating localized model training or dynamic adaptation strategies.
4. **Expanding Feature Space**: The underlying algorithms are integrating a significantly wider array of data points than historically considered, moving beyond simple structural data to include high-dimensional features like those extracted from visual assets and proxies for socio-economic context. This shift towards a broader "feature space" fundamentally changes how value signals are captured computationally.
5. **Temporal Adaptation Requirement**: Maintaining relevance in dynamic markets requires models that don't just predict based on static past patterns but can dynamically adjust. This means algorithms need to continuously learn and incorporate recent market shifts – a continuous deployment and retraining challenge from a system perspective.
6. **Data Density Dependence**: Algorithm confidence in valuations often correlates with the density of available transactional data. In markets with low liquidity or infrequent sales, the models have fewer recent examples to learn from, making robust prediction inherently more difficult and potentially less reliable.
7. **Filtering for Exception Cases**: The computational models are showing utility in identifying properties that deviate significantly from predicted norms. This capacity for 'anomaly detection' could serve as an efficient initial filter, directing human appraisers to properties that genuinely warrant deeper, non-standard investigation.
8. **Systemic Bias Risk**: A critical concern in training these models on historical datasets is the potential to embed and perpetuate biases present in past market behaviors, including historical inequities. Ensuring fairness and equity in valuations generated by these systems is a complex technical and ethical challenge requiring careful data auditing and algorithmic design.
9. **Depth of Historical Signal**: Leveraging extensive time-series data allows algorithms to potentially identify valuation factors and correlations that are subtle or emerge only over long periods, going beyond static point-in-time analysis. Mining these deep historical signals can reveal dynamics not captured by simpler methods.
10. **Aggregate Data Utility**: Beyond assessing individual properties, the aggregate data generated by these high-volume valuation systems could provide macro-level insights into market trends, urban change, and potentially inform broader planning or infrastructure decisions, demonstrating a potential utility for systems output beyond transactional needs.
More Posts from realtigence.com: